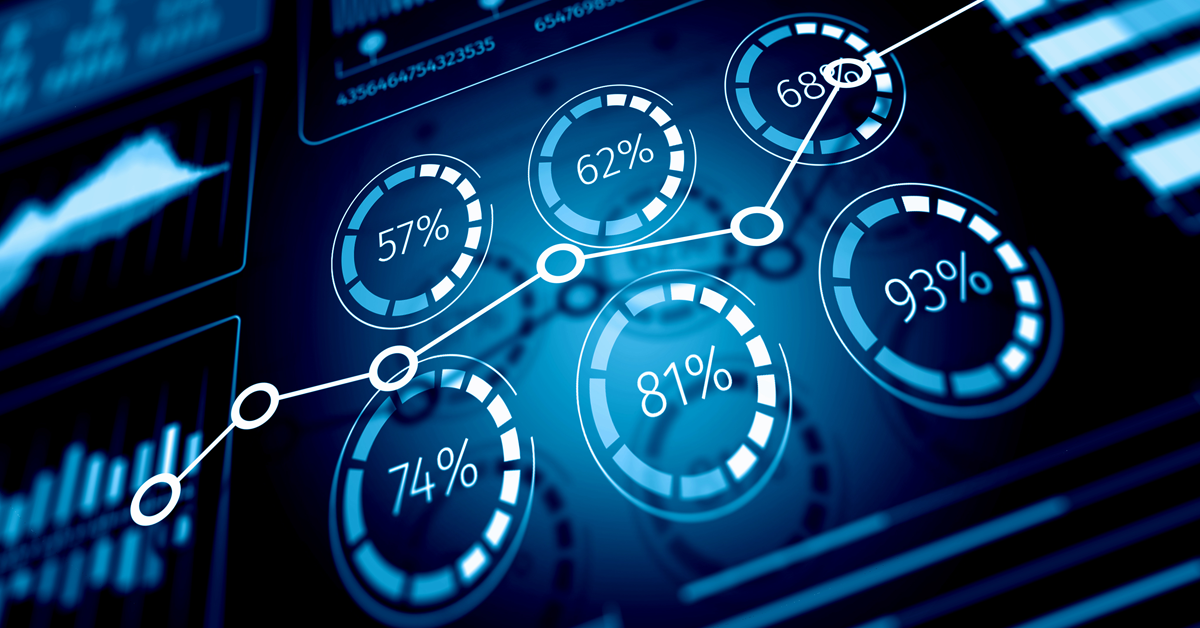
Measuring Media Investment for Success: Attribution Modeling
We know that great media performance is something very important to all of us, however one question which comes to mind is: How do we quantify and measure ‘great’ performance? The first step towards measuring for success is by being effective (capture the right data outputs), then being efficient (measure data the right way). In other words, before doing things right, we need to be doing the right things.
Over the years, there have been many ways to measure media performance, some depending on strategy, others on technical capabilities. The media ROI (return on investment) measurement has evolved from basic total revenue return (not necessarily correlated to media activations) to sophisticated data driven attribution modelling, considering all channels and touch points throughout the conversion path. The advancement of digital media technology has been tremendous, and we now have the chance to do it the right way (or shall I say, have no excuses, not to do it the right way).
Before I dive deeper into the different attribution models (ways to measure media performance), I would like to elaborate a bit on the key components that should be put in place to ensure full visibility on how well is media investment doing.
Key components:
Media channels – Enable full visibility on all media channels (link) we have invested in, regardless of the amount, time, and effort. It is important to have a 360’ view on all media activations (paid, organic, content marketing, platforms and extensions).
Touch points – Once we have the complete picture of the channels, we need to outline the consumer TPs (touch points), and clearly define and map the various CPs (conversion paths) our customers engage and do business with us.
Data layer – On the back of the CP (conversion path) mapping, we must integrate the right tagging to give us thorough information about triggers for certain customer behavior.
BI and analytics platform – With the data layer in place, we should have a BI (Business Intelligence) analytics platform to aggregate the information and visualise it in a meaningful and actionable way.
The following are some of the most common (traditional) attribution models:
Last-click attribution: This is indeed the most popular way enterprises measure media currently. In many cases this is due to lack of technology, in some it is due to lack of understanding, or perhaps both. As the name implies, all credit to the media performance is given to the last click / consumer touch point across media channels. The major flaw of this method is that it completely disregards all other channels. Depending on the type of business, the conversion path can stretch across many media activations before that last click and we need to assign the right value to all of them.
First-click attribution: Pretty much the reverse of the last-click, whereby all the revenue is attributed to the first click / touch point and all the subsequent interactions are completely taken out of the account.
Linear attribution: Using this model, the revenue is split equally among all touch points along the conversion path. This is a more balanced approach; however, it fails to rightfully capture the value of each channel towards the overall revenue.
Time decay attribution: This model gives more value to the interactions / touchpoints that happened closer to the time of the conversion. The closer the interaction is to the conversion point the more value it gets.
U-shaped attribution: The first and last clicks / touch points get the most value and the rest are allocated equal credit. Usually, the first and last interactions are each given 30% – 40% of the credit and the other 20% – 40% are allocated evenly across the middle touch points.
Choosing the right attribution model is not easy and in many cases having a complete view on all of them is very helpful. However, to avoid generalizing and prevent from over or underestimating certain channels, the best approach is a multi-facet, data driven Markov Attribution model, which in a way comprises all the above in a lot more granular manner.
Data driven (Markov) attribution: The way to apply this model is by going back to my initial point of knowing our channels and having complete view of the CPs (conversion paths). Once we understand the various CPs, we can build probability flowchart outlining each channel and their contribution towards the final conversion. Each touch point and channel are assigned a percentage contribution towards the end transaction, proportionate to their respective value, resulting in a very accurate representation of the media performance.
Having an insightful attribution modeling in place is vital to the success of our media investment, however it is just one piece of the puzzle. Simply relying on internal analysis could pose a limitation, because the key focus is predominantly within our own environment. To understand the complete picture, we should also look at external factors and analyze the overall media mix, macro, and micro variables beyond our channels. Controllable vs. non-controllable environment is a whole different topic!